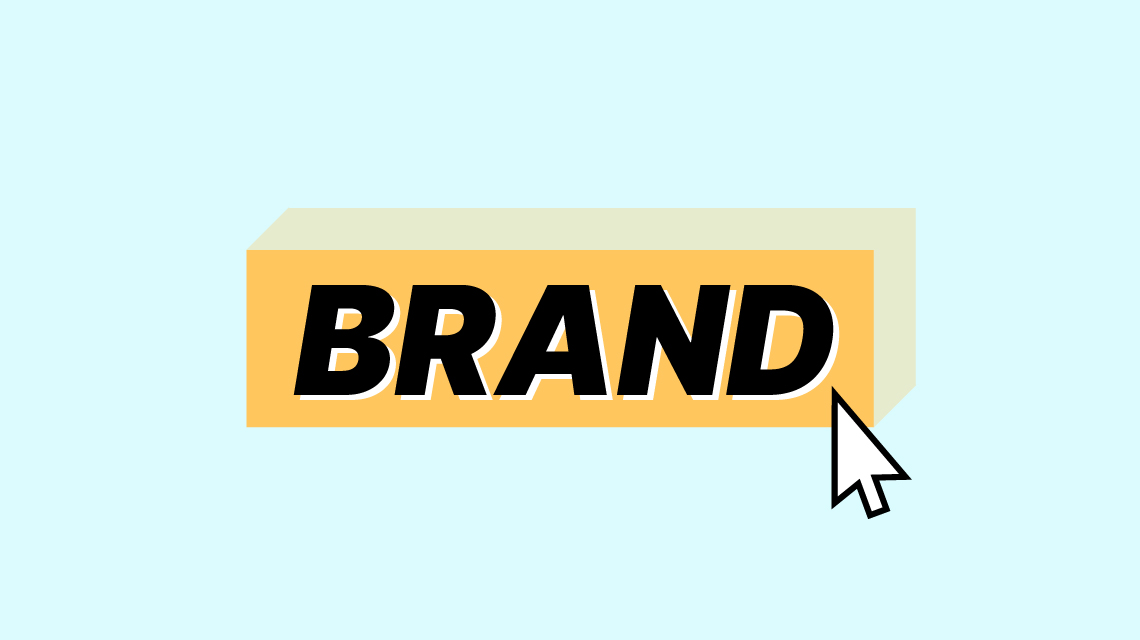
Around the globe, brands get more and more involved in big data analytics, and you can understand why. Every organization has loads of unstructured data, which is very hard to analyze but still brings many benefits.
This data is not just about numbers; the unstructured text is included in it as well. According to research, over 80% of data in organizations is estimated to consist of text. This is how text analytics became one of the big data analysis methods, which brands rely on for different purposes.
One of these purposes is to collect consumer insights and predict their behaviors. Also, data that comes from consumers is essential from the standpoint of marketing because it helps make campaigns more targeted and improve the customer-brand relationship in general.
So, today, we’ll take a look at what text analytics is, which benefits it brings, and how brands can leverage it to get valuable insights from consumers.
What is Text Analytics?
Also known as text mining, text analytics involves a large number of methodologies that have a goal of transforming big volumes of unstructured text into organized data. In other words, when doing text analytics, you’re trying to make sense of written communication that your organization has in large volumes.
According to researchers, there are seven main applications of text analytics:
- Information retrieval (IR) – text mining helps to retrieve documents with information from search engines. This application also involves keyword search.
- Document categorization (clustering) – grouping different parts of text according to different factors using different clustering methods.
- Document classification – categorizing parts of text using classification methods in text mining. These methods are based on models that use labeled examples, usually collected via machine learning.
- Web crawling – analyzing the data coming from the internet based on its interconnectedness.
- Extraction of information – finding and retrieving specific information from unstructured text.
- Natural language processing (NLP) – improving the interaction between computers and people using machine learning and natural language analysis.
- Concept extraction – uniting words and phrases into semantic groups to help find meaningful patterns in the text.
In our case, text analytics in customer experience is used to mine and evaluate the text that contains information from or about the customers. This text can come from:
- emails
- social media feedback
- surveys
- online reviews
The data from your consumers comes in large, sometimes unmanageable volumes. However, you cannot leave this data out because it often contains all the answers you’re looking for on how to improve your relationships with your customers.
Now, as you have a better idea of the benefits that text analytics can bring you in terms of consumer insights, let’s take a closer look at how you can leverage it.
1. Learn How Your Customers Really Feel About Your Brand
One of the ways how you can apply text analytics is through sentiment analysis, which is a common approach used to identify how consumers really perceive and treat your brand.
Let’s say you’ve launched a new e-commerce brand that sells custom-made reusable masks. After six months of selling products, you already have some feedback from your customers, but you want to know the general opinion of the public about your brand. Sentiment analysis can help you do exactly that by crawling the text that contains customer feedback and finding polarization within it.
Types of sentiment analysis
Depending on what your goal is, you might choose between several different types of sentiment analysis:
- Polarity precision. Also known as fine-grained sentiment analysis, this approach describes our hypothetical situation above and aims at helping you find polarization in public opinion regarding your brand.
- Detection of emotions. This approach aims more towards identifying certain patterns in consumer behavior. It involves natural language processing and detects emotions based on the systems of lexicons, or words that define certain emotions. This method, however, cannot be 100% precise because people express their emotions in different ways.
- Aspect-based analysis. This type of sentiment analysis allows you to pick a certain feature of your product and crawl the text to see how consumers feel about it. While the first two approaches are more general, this one can help you get customer opinion about separate characteristics of your product and use this information to improve your product or market it more effectively.
Interestingly enough, brands can benefit from sentiment analysis to not only find out public opinion about their products but also can involve this approach in competitor analysis.
For instance, let’s say you own a content marketing agency, and you want to open a new online writing service that will connect professional writers and your clients, but you hesitate whether it is a viable idea.
In this case, you can use sentiment analysis to crawl the opinions about similar services offered by your competitors to see whether their customers are satisfied. Besides, the data mined from consumer feedback will also give you ideas on how to create a service that will have the features that your competitors lack.
2. Incorporate Text Mining into Social Data Analysis
A bit earlier, we mentioned that, in terms of collecting consumers, text analytics is often used to crawl user feedback on social media. Let’s dwell on this topic a little bit more.
Marketers love social media not just for the opportunity to raise brand awareness but also for all the social data that they can collect from these platforms.
Social data involves information that is publicly shared by users, mostly on social media. This data brings a lot of useful insights and can be involved in different stages of a marketing campaign.
For example, marketers can use social data to get a better definition of the target audience persona as it often contains information on the follower demographic. Brands can see this information come in real-time via the Analytics tabs on respective social platforms. Here’s how this data looks on Facebook:
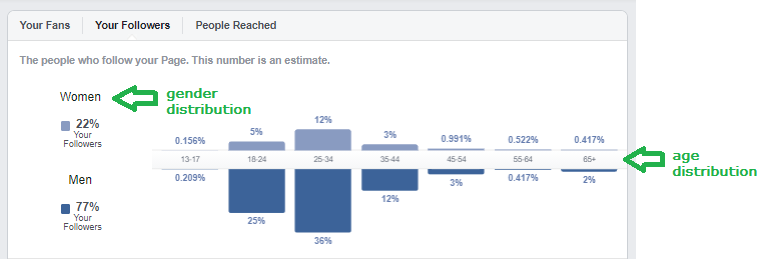
Besides developing a target audience persona, marketers often collect and analyze social media data to find out content preferences, which are crucial for launching a successful social media marketing campaign.
For this purpose, brands can also browse the Analytics tab to find the reach and engagement of each post and deduce the types of content that could potentially perform well during the campaign:
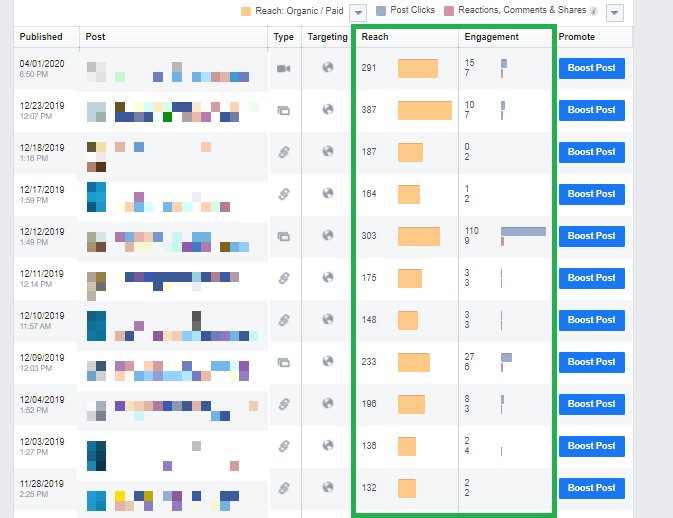
However, this information might not be enough to define the ultimate list of content types for your social media marketing campaign. This information is mostly collected from likes, shares, as well as content views. However, social data also includes other types of user feedback, including comments, which consist of text.
Social data provides a lot of valuable insights, but users don’t always share their feedback in one particular way. Some of them may simply like or share your post, while others would prefer to leave a comment. That’s why you need to involve text analytics to have a better understanding, what your followers like and dislike about your content strategy.
Surely, analyzing all the comments from your followers by hand is inefficient. You need a proper tool that will not only get all the social data centralized but will also do social listening and deliver deeper consumer insights.
If you’re on the hunt for such a tool, quintly might be a great option for you. We offer brands a lot of social media analytics solutions, from analyzing and keeping the data from all your social media accounts together in one place to getting access to public and private data for all your social channels and crawling all types of feedback coming from your followers.
3. Use Text Analytics to Identify Customer Pain Points
Lastly, doing text analytics can help you determine the biggest challenges that your customers face when interacting with your brand or using your product. These insights are very valuable and sometimes can even impact and change your marketing efforts.
In the case of identifying customer pain points, brands usually have to deal with analyzing negative feedback. To do that successfully, you will need to apply the methods of natural language processing on each of the following steps:
- Create a list of trigger words. Start with the list of words and phrases that you think might describe general frustrations your customers have with your product or brand in general.
- Set the alarm for these triggers. Use your analytics software to notify you each time when your brand is mentioned in a customer comment that uses words and phrases from the list.
- Analyze the collected results. Once you have unstructured text with customer feedback, you can start an aspect-based analysis to pinpoint the most common issues your customers have with your product.
Here, it is also important to note that not all negative customer feedback should be taken into consideration. When you already have the text analysis, try to focus on constructive feedback that has led to a customer unsubscribing, a product return, etc. This will help you identify the real concerns that consumers have with your brand and separate it from unconstructive negative comments.
Final Thoughts
It’s hard to underestimate the benefits that text analytics can bring you in terms of consumer insights. People are quick to share their feedback on the internet, and you cannot miss the opportunity to use their comments to improve your customer-brand relationships.
You can involve text analytics in everything – to find polarizing opinions about your brand, see how your customers really feel about your product, improve your social media marketing efforts, and even identify customer pain points.
However, to get all these benefits, you need a good software that will help you organize loads of unstructured text. At quintly, we will gladly help you start incorporating text analytics and get the most out of consumer insights.
Join the conversation. Leave us a comment below!